Educating artificial brains to drive autonomously: Real-world training data by human-free annotation
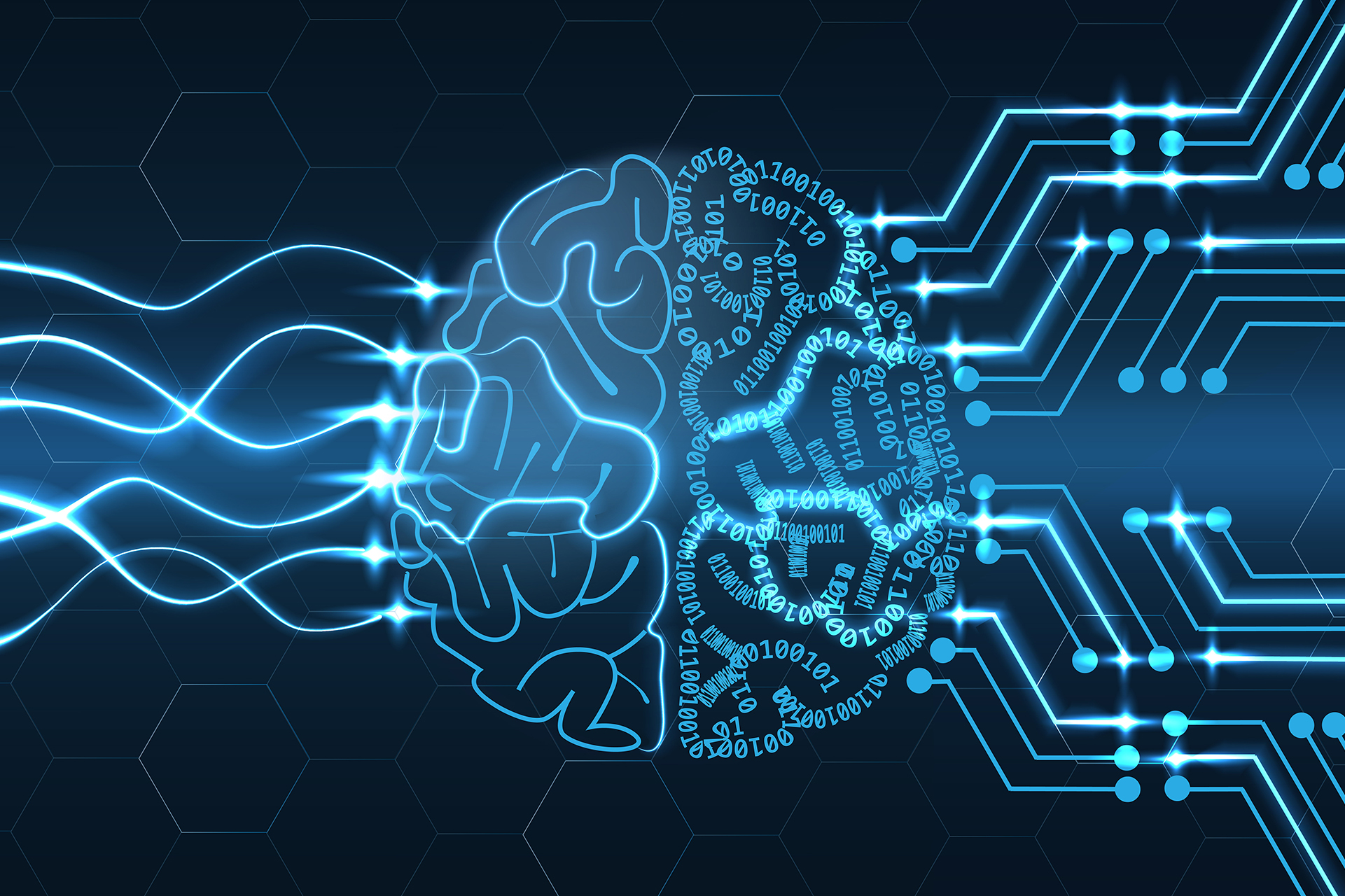
AI kills
AI can kill if given tasks beyond its intelligence "“ or beyond the level of training it received to do its job. The case of the poor woman run down by an autonomous Uber vehicle in Tampa, Arizona, in March this year, is still fresh in everyone's minds. Much speculation arose as it what caused arguably the first human casualty at the hands, er, wheels of AI. Just recently, in late May, the US National Transportation Safety Board released a preliminary investigation report, which boils down to a succinct headline, "the sensors worked; the software utterly failed." As if driving with the emergency braking disabled wasn't bad enough, the report paints a damning picture of the object detection performance by the vehicle's environmental perception AI:
"As the vehicle and pedestrian paths converged, the self-driving system software classified the pedestrian as an unknown object, as a vehicle, and then as a bicycle with varying expectations of future travel path," the report says.
This goes to show how important the classification of objects is, because each object class has its own properties that determine its possible travel paths. In reality, an autonomous vehicle must be able to detect and correctly classify each and every object present in a complex scene. In AI / computer vision jargon, this is known as instance-level object understanding, which dictates that all instances of a class (e.g., cars) must be detected, located and identified in an image produced by the specific imaging sensors (cameras and LiDARs) mounted on the vehicle.
Why training matters
So what was wrong with Uber's image understanding AI? The short answer is, training, or rather, lack thereof.
Environmental perception of autonomous vehicles is powered by deep neural networks that need hundreds of thousands, if not millions, of examples to learn the appearance of common and not-so-common objects that they could possibly encounter in real-world traffic. For supervised machine learning, you need lots of annotated, or labelled data, meaning an image with object labels and the precise pixels occupied by those objects. The labelling of the different objects and areas in an image by class is referred to as semantic segmentation, and the labels and corresponding object locations as "ground truth." Large, diverse, high-fidelity training datasets are essential to autonomous vehicles using deep learning for scene understanding.
Fig.1. Object detection by a state-of-the-art neural network.
The coloured shapes in Fig.1 show the objects recognised by a state-of-the-art neural network, which failed to detect a particular van "“ perhaps because it blends in with the road surface. So this network needs to go "back to school" for further training to learn what vans look like. The same goes for Uber's AI, which needs to be schooled with a few thousand more images of pedestrians crossing a road at night. And do so through the eyes of specific sensors on the vehicle "“ visible light and night-vision cameras and LiDARs, so training datasets are needed for all these different imaging modalities. Then through sensor fusion one can combine the strengths of the different sensors to build an accurate worldview around the vehicle.
AI training datasets
How is AI training done today? Neural networks are usually pretrained on synthetic data, which are obtained from VR simulators of proxy worlds driving virtual cars. This approach entails significant 3D modelling effort to build proxy worlds and lacks both photo-realism and diversity. Actually, one can get very photo-realistic scenes that almost look like the real thing as in Fig.2 (left). Almost, but not quite "“ which why one needs to complete the training on real-world data that contain low-level detail and physical sensor effects. Real-world data contain sensor bias (e.g., aberration, blur, exposure, noise) but require manual human labelling to generate "ground truth," which is tedious and slow (up to 1 hour per image). The preparation of hand-labelled data as in Fig.2 (right) is usually outsourced to cheap labour countries like India and Bangladesh. Still, labelled real-world data is very expensive and costs up to $10 per segmented image.
Both the synthetic and real-world data have their pros and cons, and are used in a complementary fashion.
Fig.2. Images from synthetic (left) and real-world, hand-labelled (right) training datasets.
STRADA datasets
Enter TheWhollySee, a nascent start-up from the Holy Land, er.... Israel, on a mission to build training datasets for different types of imaging sensors on an autonomous vehicle.
At TheWhollySee, we are developing a breakthrough technology, which we call STRADA, that takes the best of the synthetic and hand-labelled data approaches. It involves automatic augmentation of real-world data with the use of our proprietary imaging system. We can generate high-fidelity, high-diversity augmented real-world data that does not require the expensive human labelling or post-processing. By augmenting real-world imagery in multiple sensor modalities, we can achieve greater realism and diversity than synthetic data from computer-generated proxy worlds.
Fig.3. Dataset product portfolio under development at TheWhollySee.
While our technology is still under development and we are not yet in a position to offer datasets, we have a plan for building tailored data packages for different types of customer as shown in Fig.3. For example, we can build targeted foreground datasets to capture new vehicle models that will hit the road in the next couple of years and need to be correctly detected and recognised by other autonomous users.
The second type of data is focused on different backgrounds that represent the environments where autonomous vehicles need to operate. These could be specific settings such as airports and campuses, or broader country-specific backgrounds like local countryside scenes.
Next, we can build reinforcement datasets for autonomous buses and shuttles to increase the recognition accuracy along specific routes. When a bus travels the same route thousands of times over and accumulates the risk in doing so, the AI brain of the bus must make absolutely no mistakes (e.g. missed detections or false positives) all along the route.
Finally, the safety of autonomous agents is predicated on their flawless environment sensing and perception, which require verification and certification using their sensor-specific data. However, the scene diversity and variety of edge cases achievable with physically driveable mileage are insufficient for fully autonomous driving certification. To address this need, we will generate a variety of edge cases and scenarios to test the perception function of autonomous agents. With our data we can count the number of missed detections and false positives to verify and certify the safety of driverless operation.
We are actively seeking both customer feedback and venture funding to build these training and certification datasets that will ultimately guarantee the safety of autonomous driving "“ and make the Uber incident a non-recurring singularity of the past.
Educating artificial brains to drive autonomously: Real-world training data by human-free annotation
Modified on Friday 10th August 2018
Find all articles related to:
Educating artificial brains to drive autonomously: Real-world training data by human-free annotation